Born of silicon and starlight (009)
In the silence of the byte / A dance of codes, so terse and tight / Gives the machine the gift of sight.
Welcome to Artificial Insights – your weekly review of links to articles, interviews and memes helping you understand our transition towards more kinds of intelligence.
Two months into publication, the opening rates and clickthroughs on Substack have been surprisingly consistent: a whopping 55% view each edition, of which circa 10% end up clicking on a link – which tells me 90% of you are here for the commentary (!).
This week’s edition features the best of what has populated by YouTube and Twitter reccomendation algorithms lately. I am spending all free time learning about as many perspectives as possible when it comes to our transition towards AGI, and this newsletter attempts to highlight and translate the most important ideas and voices as they come across my feeds.
If this resonates, consider sharing this week’s edition with friends & enemies on your socials to help us grow and reach more likeminded people looking to learn. AI will change everything – it already is – so let’s make sure the right voices are part of the conversation.
This week’s edition features a handful of new subheaders and format adjustments. Send feedback and memes my way!
MZ
Virtual symposium about AI and climate change 🌊
AI might be used to optimize complex systems and improve predictions, which could help address the climate crisis. But it also features a significant carbon footprint and externalities when it comes to training data and human resources. The Climate Change AI initiative recently offered a Summer School lecture series (3h) on YouTube with several speakers addressing the topic from a variety of perspectives. Hard to summarize but my highlight was Lyyn Kaack’s rather technical outlook on the field and Dr. Kamal Kapadia’s definitions of social justice for AI. I also liked how all speakers raised the need for both individual and institutional change.
On technological revolutions and continuous exponentials 👀
Sam Altman is back from a global tour with leaders and policy makers and shares some insights on a 20-min interview with Bloomberg Live. The whole thing is worth a listen but most of it revolves around the learnings from diverse stories and the increased importance for global coordination and regulation. Altman is asked whether OpenAI is calling for regulation in public but arguing for deregulation in private – and his reasoning around regulatory capture was fascinating to me. He briefly talks about “AIs designing other AIs” which is probably how GPT-4+ is being trained. He also explains why he has no equity in OpenAI, and sees AI as the next crucial step for humanity to solve unsolved problems.
On the emerging tasker underclass 🛟
Investigative long read from Josh Dzieza on New York Magazine / The Verge about some of the less know and understood aspects of AI development: the labor exploitation and low-paid bullshit jobs that inevitably are needed to support the creation and maintenance of our AI tools, like annotating photos for learning systems.
It’s a strange mental space to inhabit, doing your best to follow nonsensical but rigorous rules, like taking a standardized test while on hallucinogens.
Trillion Dollar Questions ♟️
Marc Andreesen on Lex (3h) talk alignment and possible futures. Profound and fascinating. Highly worth a listen – the conversation ranges from the immortality of LLMs, what it means to jailbreak AGI, what it means to forget after AI, whether our interactions with AIs (RLHF) are creating fresh sources of signal, what it means to debias content (pulling out emotionality via AIs) and so much more. Dense and insightful and highly likely – especially Mark’s predictions about how these assistants will be embodied and incorporated into our lifes and regulatory capture. For more, check out his recent essay.
Hallucination is what we call it when we don’t like it - Creativity is what we call it when we do like it.
Shorts
GPT-4 might be eight smaller specialized models stacked together, as opposed to one massive model. Soumith Chintala and Geohotz on Twitter figure OpenAI could have coupled multiple smaller models in order to maximize efficiency to achieve a trillion parameters. 👶🏽👶🏽👶🏽🧥
Harvard’s new computer science teacher is a chatbot. Students enrolled in the university’s flagship CS50 course will be presented with the AI teacher in September.
Paper by Singapore University of Technology and Quebec AI Institute inadvertedly reveals why AI is set to replace humans at many jobs:
The figure shows that the number of misclassifications decreased even further after removing the evaluations of human raters who did not scroll to read the full explanations.
Humans get tired without realizing it – AI never gets tired / bored with a task.
Learning AI
The Institute For The Future (IFTF) is offering a paid training course on applied AI and foresight called Foresight Essentials Course — Three Horizons of AI ($950). Live and online.
Sebastian Raschka (PhD) launched a book with hands-on exercises called Machine Learning Q and AI ($30). Also on Substack.
Article by Matt Might called Guide to Perceptrons (via HN).
Prompt Engineering Guide (via Fabio).
AI Glossary by a16z – from accelerator to zero-shot learning.
Frequently Asked Questions by Stanford professor John McCarthy about AI.
I don't see that human intelligence is something that humans can never understand.
Using AI
Board of Innovation – AI Powered Innovation Sprint.
DesignSparks – Explore new perspectives and possibilities for your design process through AI generated sparks.
Generative AI to create more powerful tools and futures narratives by Torchbox.
We followed a 20:60:20 or “machines in the middle” principle ensuring humans were always in the loop.
20% - Us. We crafted initial prompts with clear instructions on the context, including the desired timeframe and early iterations of our stories from the activity.
60% - GPT-4 or Claude did much of the heavy lifting with guidance from us.
20% - We refined and further crafted the outputs of the content
Long Reads from Substack
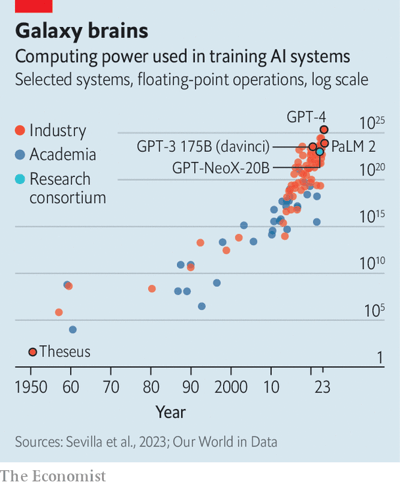
Emerging Vocabulary
Inference
The process of using a trained machine learning model to make a prediction or decision. It's the stage where the model, after being trained on a large amount of data, applies what it has learned to new, unseen data. In many real-world applications, inference needs to be fast because it often happens in real-time. For example, in autonomous vehicles, the machine learning model needs to infer the objects around the vehicle using sensor data in real time to make driving decisions.
View all emerging vocabulary entries →
Project Showcase
Factiverse.ai
Factiverse uses advanced machine learning to analyze text and identify factual statements. It then provides live sources that either support or dispute those statements. It is trained on articles from reputable news media and certified fact-checkers.
AI Tools Used
Summarize.tech to summarize YouTube links.
ChatGPT wrote the subject line poem about AI and summarized articles.
If Artificial Insights makes sense to you, please help us out by:
Subscribing to the weekly newsletter on Substack.
Following the weekly newsletter on LinkedIn.
Forwarding this issue to colleagues and friends wanting to learn about AGI.
Sharing the newsletter on your socials.
Commenting with your favorite talks and thinkers.
Artificial Insights is written by Michell Zappa, CEO and founder of Envisioning, a technology research institute.
You are receiving this newsletter because you signed up on envisioning.io.